Learn how demand planning and forecasting help you optimize inventory, meet customer needs, and boost profitability by predicting and preparing for future demand.
Home » Data Engineering
ETL transformation types include processes like data cleaning, filtering, aggregation, enrichment, and joining. These transformations prepare raw data for seamless integration and actionable insights.
Explore essential data engineering best strategies to design scalable, efficient data systems that ensure seamless integration, optimized performance, and future-proof architecture.
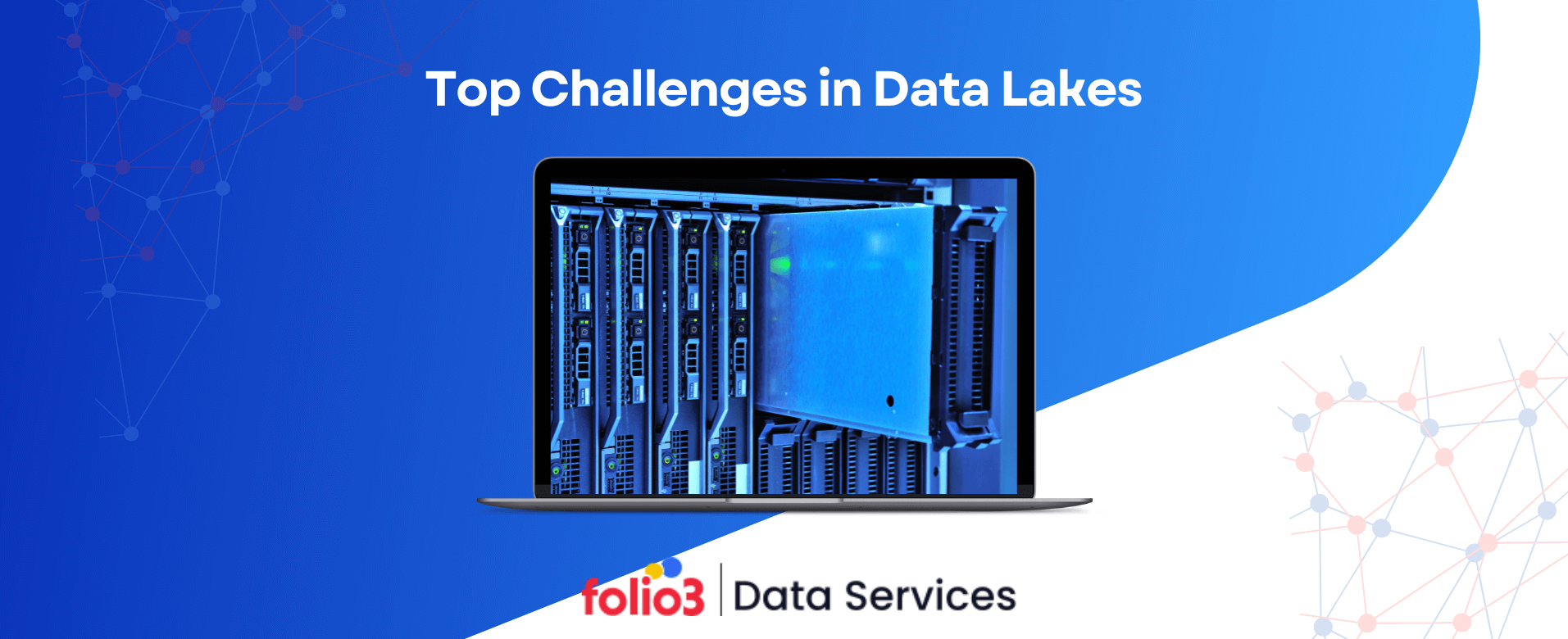
Organizations face challenges with data lakes, including handling large data volumes, maintaining accuracy, ensuring proper governance, integrating systems seamlessly, and managing storage efficiently.
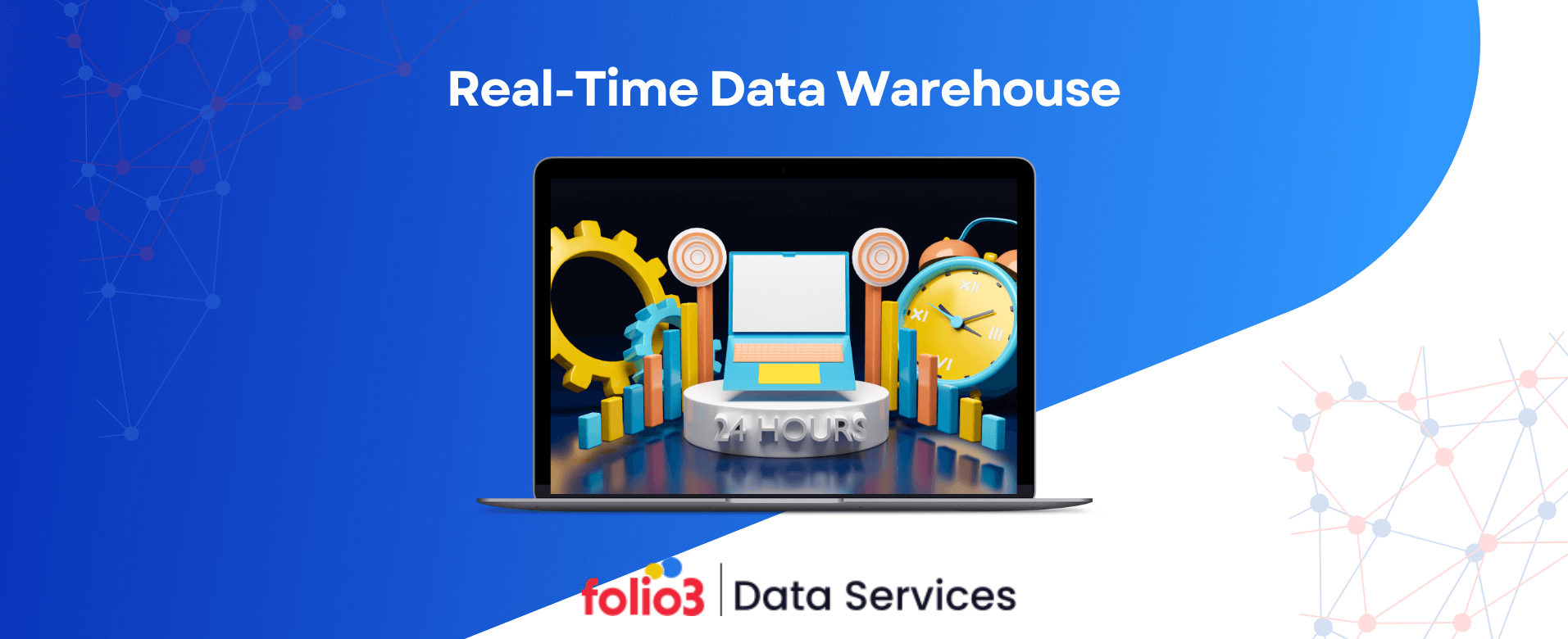
Data-driven decisions are critical for success in staying in a top business environment. However, traditional data warehousing methods often need to improve to deliver the
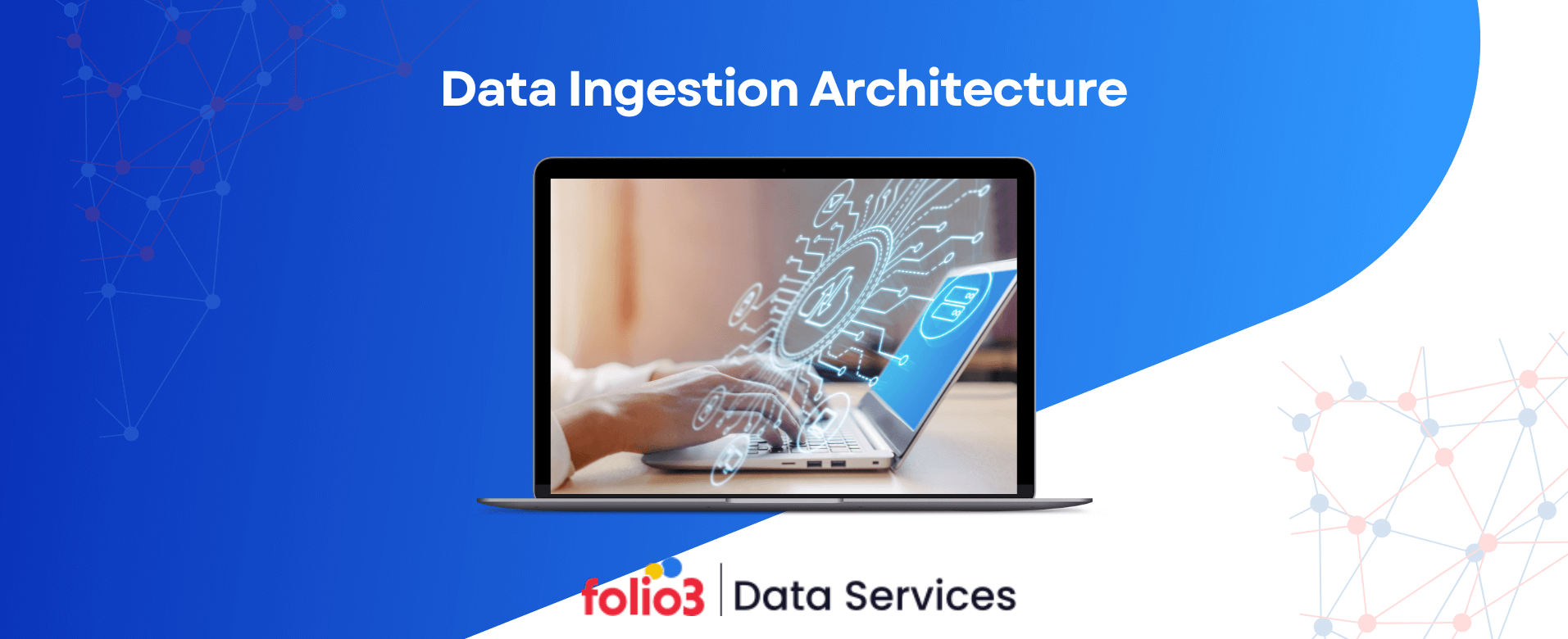
Delve into the critical design principles, advanced tools, and proven best practices that form the foundation of a robust data ingestion architecture, enabling seamless data flow, scalability, fault tolerance, and enhanced data quality for analytics and decision-making.
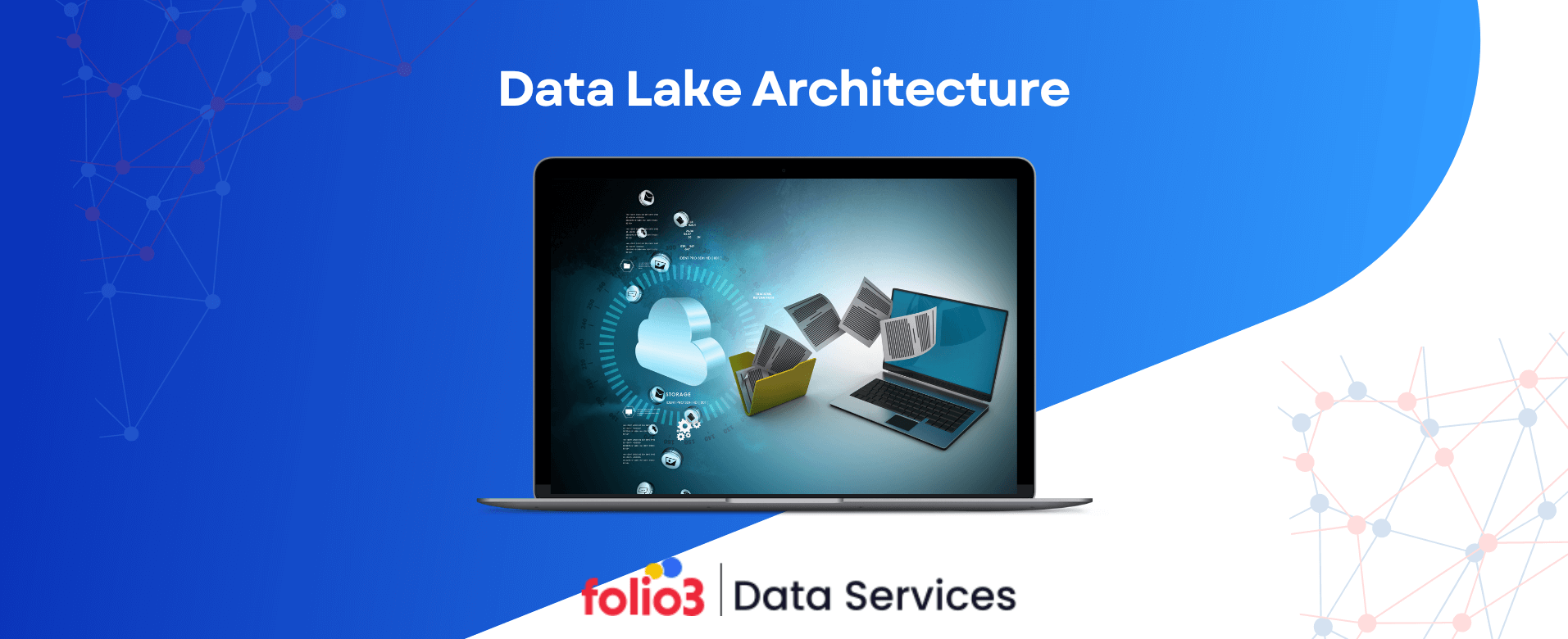
Businesses across industries are generating vast amounts of data. Whether structured, semi-structured, or unstructured, this data holds immense potential for gaining insights and driving innovation.
Discover how this transition offers unique benefits while addressing essential considerations and challenges.
Methods for unifying data from multiple sources include ETL (Extract, Transform, Load), ELT (Extract, Load, Transform), real-time access through data virtualization, unified querying with data federation, and continuous syncing via change data capture (CDC).
Dive into the fundamental components and architectural design of a big data pipeline, detailing each stage from data ingestion to processing, storage, and analysis, enabling efficient and scalable data workflows.